Revolutionizing Business with Data Labeling in Machine Learning
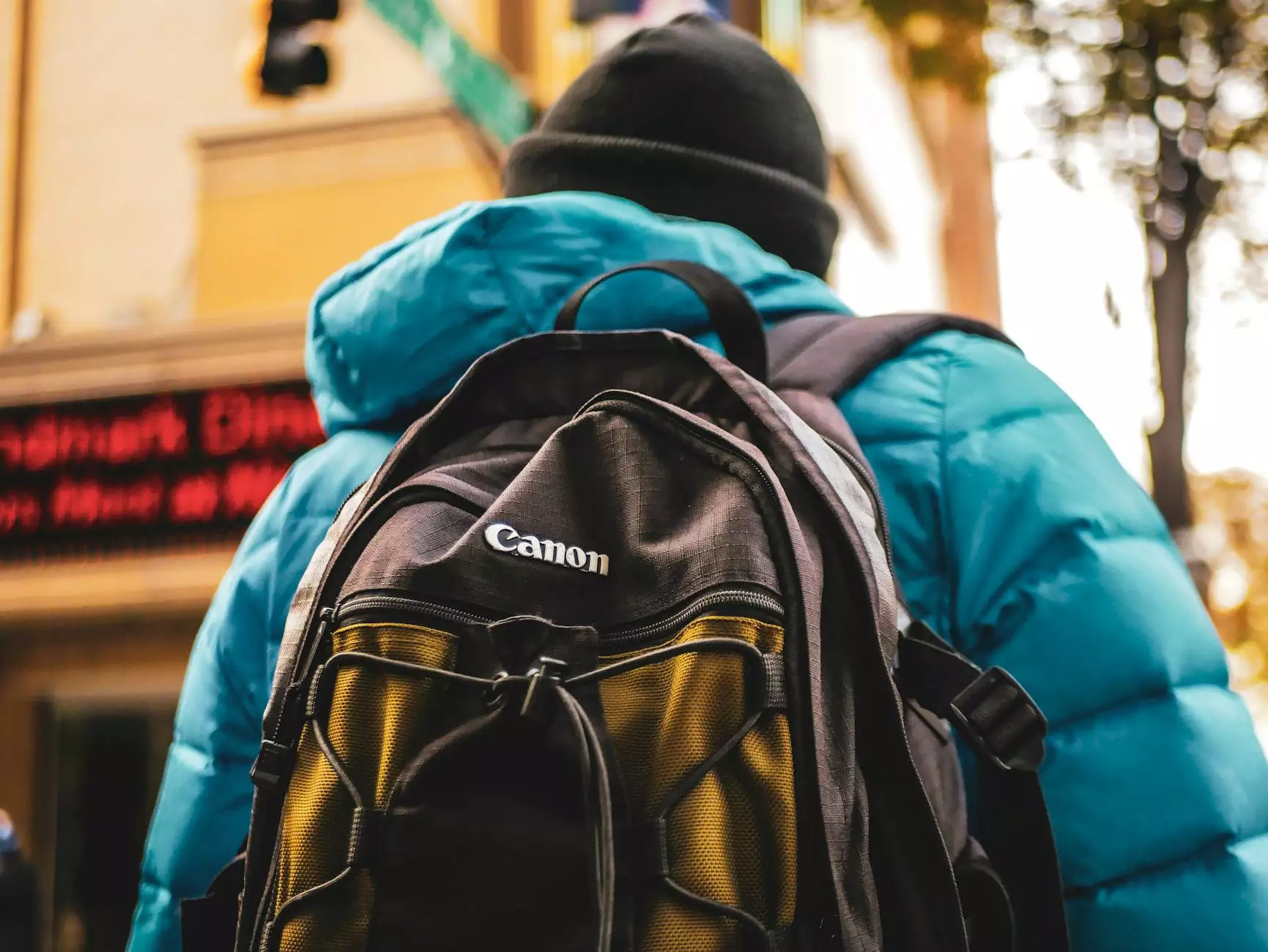
In an era where data drives decisions, proper management and utilization of data are more critical than ever. Companies across various industries are recognizing the potential of data labeling machine learning as a tool to refine and optimize their processes. As we delve into this transformative topic, we will explore the importance of data annotation, the advantages of using dedicated platforms like KeyLabs, and how a systematic labeling approach can propel a business forward.
The Necessity of Data Annotation in Machine Learning
Data is often referred to as the new oil, an asset with immense value when refined correctly. However, raw data is often unstructured and devoid of meaningful insights. This is where data labeling comes into play, particularly in the context of machine learning. To train machine learning models effectively, large volumes of data set as input must be accurately labeled.
- Understanding Data Labeling: Data labeling is the process of assigning informative tags or labels to raw data. This can be images, text, audio, or video, allowing machine learning algorithms to learn from these datasets effectively.
- Machine Learning Models and Data Quality: The efficacy of a machine learning model is directly proportional to the quality of the labeled data used for training. High-quality labels facilitate better pattern recognition, leading to more accurate predictions.
Key Functions of a Data Annotation Tool
A robust data annotation tool serves various functions that are essential for businesses aiming to harness the power of machine learning. Here are some key features that such platforms typically offer:
1. Variety of Annotation Types
Data labeling encompasses diverse forms of annotations based on the type of data being processed:
- Image Annotation: This includes bounding boxes, polygons, and semantic segmentation for image recognition tasks.
- Text Annotation: Tagging and categorizing text for natural language processing tasks, ensuring sentiment analysis and entity recognition.
- Audio Annotation: Transcribing spoken language or labeling audio segments for speech recognition technologies.
2. Collaboration and Workflow Management
Efficient data annotation platforms facilitate seamless collaboration amongst teams. These tools offer:
- Real-Time Collaboration: Multiple users can annotate simultaneously, improving efficiency and speeding up project timelines.
- Task Management Features: Monitor the progress of various annotation tasks, making it easier for managers to allocate resources effectively.
3. Quality Assurance Mechanisms
Ensuring the accuracy of labeled data is pivotal. Many platforms integrate features such as:
- Review and Feedback Loops: Annotated data can be reviewed by experts, ensuring high standards.
- Automated Quality Checks: Some platforms employ algorithms to detect inconsistencies or errors in annotations, streamlining correction processes.
Advantages of a Dedicated Data Annotation Platform
Investing in a specialized data annotation platform like KeyLabs offers numerous advantages that can greatly benefit businesses:
1. Increased Operational Efficiency
By outsourcing data labeling tasks to dedicated platforms, companies can redirect their internal resources towards core business functions. This leads to significant improvements in operational efficiency.
2. Enhanced Model Accuracy
The more refined and accurate the data annotations are, the higher the accuracy of the resulting machine learning models. This leads to superior product offerings and customer satisfaction.
3. Scalability
As businesses grow, so does the volume of data they handle. Advanced data annotation platforms are designed to scale according to the needs of the business, ensuring that the data annotation process keeps pace with growth.
Implementing Data Labeling for Business Success
Utilizing data labeling machine learning in business requires strategic implementation. Here are some crucial steps that organizations should follow:
1. Define Goals and Objectives
Before embarking on a data labeling project, organizations must outline their goals. Whether it's to enhance product features, improve customer service, or develop new AI-driven solutions, clarity in objectives is crucial.
2. Choose the Right Data Annotation Tool
Select a data annotation tool that aligns with your needs. Evaluate platforms on the basis of:
- Usability: It should be user-friendly and intuitive.
- Customization Options: Different projects may require different styles of labeling.
- Integration Capabilities: The tool should seamlessly integrate with your existing systems.
3. Train Your Team
Your team should be well-trained in utilizing the data annotation platform. Conduct training sessions to ensure everyone is capable of contributing effectively to the annotation process.
4. Monitor and Evaluate
Once your data labeling project kicks off, it's critical to monitor the progress and evaluate the quality of the annotations regularly. Adjust strategies as necessary to maintain high standards.
Case Studies: Successful Implementation of Data Labeling
Many companies have experienced transformative results by implementing effective data labeling practices. Let’s discuss a few examples:
1. Healthcare Sector
In the healthcare industry, machine learning models require accurate labeling of medical images for diagnostics. A leading healthcare provider integrated a data annotation platform to label thousands of images used in developing algorithms capable of detecting diseases like cancer. The result was a significant decrease in diagnostic errors, showcasing the critical role of high-quality annotated data in healthcare.
2. E-commerce Industry
An e-commerce giant implemented NLP models with labeled data to better understand customer sentiment and intention. By analyzing customer reviews and feedback effectively, the business was able to tailor its marketing strategies, improving customer engagement and increasing sales by 25%.
3. Autonomous Vehicles
Automotive companies are utilizing data labeling for training autonomous vehicle systems. By labeling various elements in driving scenarios, including pedestrians, signs, and other vehicles, manufacturers improve the machine learning algorithms that power self-driving cars, enhancing safety and reliability on the roads.
Conclusion
The significance of data labeling machine learning cannot be overstated. As businesses increasingly leverage machine learning to innovate and streamline their operations, the demand for high-quality, accurately labeled data will only continue to grow. Companies that invest in high-end data annotation tools and platforms will undoubtedly enjoy a competitive edge in their respective industries.
Platforms such as KeyLabs not only provide advanced data annotation tools but also ensure that businesses can scale their data operations efficiently while maintaining quality. The path to data excellence begins with proper labeling, setting the foundation for future successes in an increasingly data-driven world.